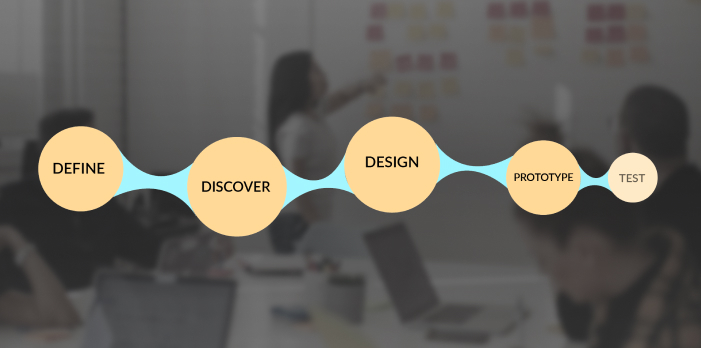
Over the past few months, Panorama Innovation’s internal team has been exploring the crossroads of the design process and Artificial Intelligence (AI). In this article, we delve into the transformative landscape of how the rapidly evolving landscape of Artificial Intelligence impacts the foundation of design processes. We welcome comments and discussion about navigating the ever-changing currents of this dynamic field to unlock new insights for the future of design.
How can Artificial Intelligence and diverse language models be utilized to produce solutions impacting human systems? In what ways do AI platforms and tools enhance traditional design processes? How does AI influence human cognition, work patterns, and lifestyles? These are a few of the many questions that guided our inquiry.
In the dynamic landscape of technological advancements, Artificial Intelligence is here to stay. Within design research, in particular, it is shaping the way user data is collected, interpreted, and communicated. It can surface valuable data, filter out unnecessary information, generate insights, and craft meticulous documents. We explored the emergence of AI and focused on understanding the extent to which design processes could be automated; identifying areas where human intervention remains crucial.
Applications in Design Research
For this project, we focused on AI tools that are used to analyze and synthesize user data gathered through primary research.
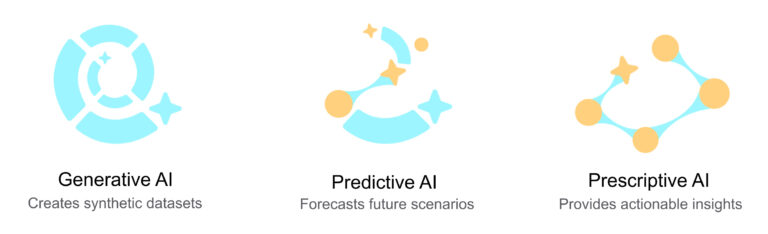
These Large Language Models (LLMs) can be integrated into the human-centered design process to aid the workflow. Amidst these categories, Generative AI transforms content creation from personalized products to synthetic datasets. Predictive AI enables businesses to foresee and optimize future scenarios, offering insights into customer behavior and market demands. Meanwhile, Prescriptive AI takes it a step further, providing actionable recommendations for optimal outcomes in healthcare, customer retention, and logistics. This project focused on analyzing Prescriptive AI as a tool for design research.
Visualizing the Human-Centered Design Process Enhanced by AI (Click to enlarge)
Tools and Takeaways
In today’s market, an array of prescriptive AI tools cater to different facets of the research process, with new ones emerging regularly. Our objective was to identify and assess a platform that would streamline interview transcription, data analysis, pattern identification, and overall data comprehension. For this study, we looked at ChatGPT, Notably, Marvin AI, Kraftful, UserDoc, Pendo, Bard, OutsetAI, Recollective, Grain, and Dovetail.
What worked?
Several of the AI tools swiftly transcribe audio and video, creating accurate, concise summaries from interviews. They can intelligently filter data, tag participants for organized quotes, and automate large text analysis, offering intuitive real-time assistance as well as responsive customer support with timely updates.
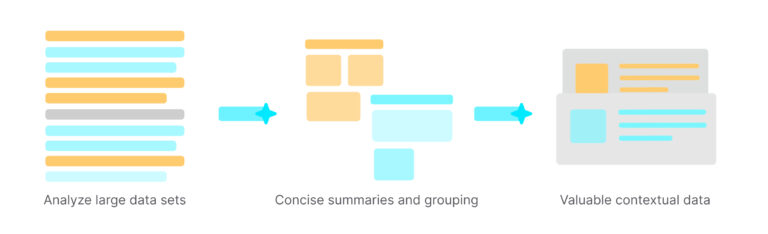
Here is a summary of general strengths:
- Quick transcription of video and audio data.
- Concise summaries derived from interview transcripts.
- Easy to filter data based on directional context.
- Quick tagging for organized clustering of quotes from various sources.
- Conversion of data into insights presented in a communicable format.
- Automation of analyzing large text volumes, forming a distinct point of view.
What didn’t?
Issues regarding confidentiality and data ownership arise, despite various tools’ capabilities in transcription, pattern finding, clustering, and sensemaking. The tools we reviewed relied on participant-provided information and could not observe behavior beyond that explicitly shared information for insight generation.
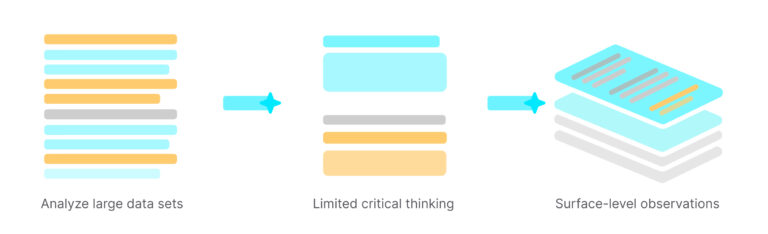
Here are some of the drawbacks of AI tools:
- Confidentiality and data ownership is a concern. Many companies are reluctant to use even closed-source alternatives to analyze their customer data.
- Themes and patterns identified by AI analysis may not be relevant to the study. Needs human review to ascertain the significance and importance of findings.
- Sole reliance on what the participant ‘says’. Absence of observational capabilities, resulting in a surface-level baseline for insight generation.
- Lack of ability to read between the lines or ask follow-up questions to the participant if it is an AI-led interview.
- Recognition is limited to data generated by AI, and is dependent on accurate tagging.
Exploring AI’s impact on research methodologies, the following insights offer a glimpse into its transformative potential and limitations.
AI is…
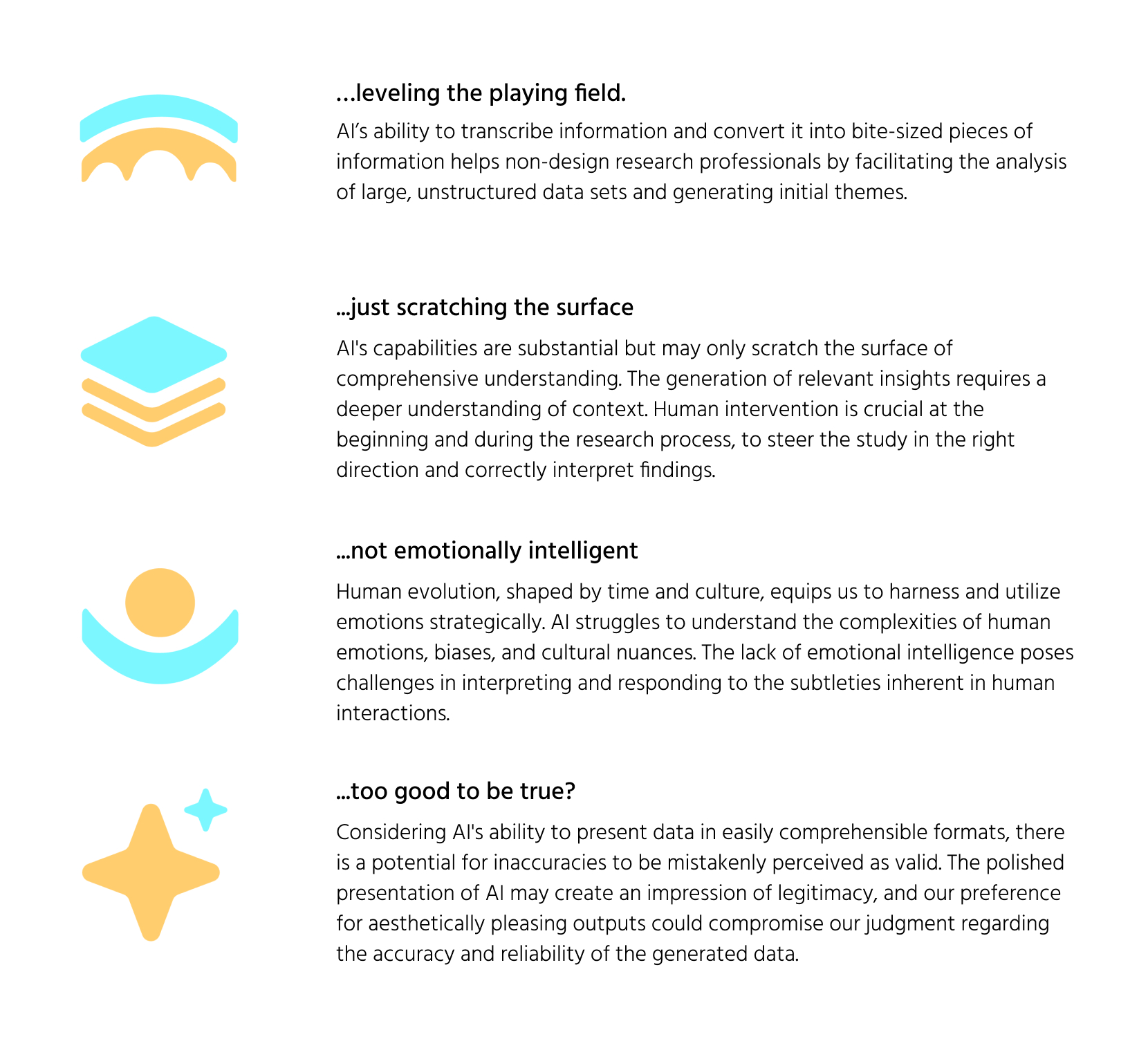
Embracing AI as a Team Member
In the evolving landscape, redefining our perspective on AI is imperative. Rather than viewing it as a mere tool or technology poised to supplant human roles, we should embrace it as a collaborative team member. This paradigm shift entails optimizing our collaboration with AI and adapting our methodologies to achieve optimal results aligned with the overarching project goals. This could involve a nuanced comprehension of AI capabilities, assigning tasks accordingly, and discerning moments where granting it autonomy is beneficial, balanced with instances requiring human intervention. Establishing comprehensive guidelines that underscore the authentic integration of AI across diverse contexts becomes essential.
References
Generative, Predictive, Prescriptive AI: What They Mean For Business Applications – Bernard Marr— https://bernardmarr.com/generative-predictive-prescriptive-ai-what-they-mean-for-business-applications/
What are the 3 types of AI? A guide to narrow, general, and super artificial intelligence— https://codebots.com/artificial-intelligence/the-3-types-of-ai-is-the-third-even-possible